Sentiment (Discover)
About Sentiment
Sentiment is an enrichment provided to you in all of your XM Discover data sources. Sentiment measures how positive or negative an individual comment is. Sentiment is calculated at a sentence level on a -5 to 5 scale, and can be divided into either 3 or 5 scale segments: Very Negative, Negative, Neutral, Positive, and Very Positive.
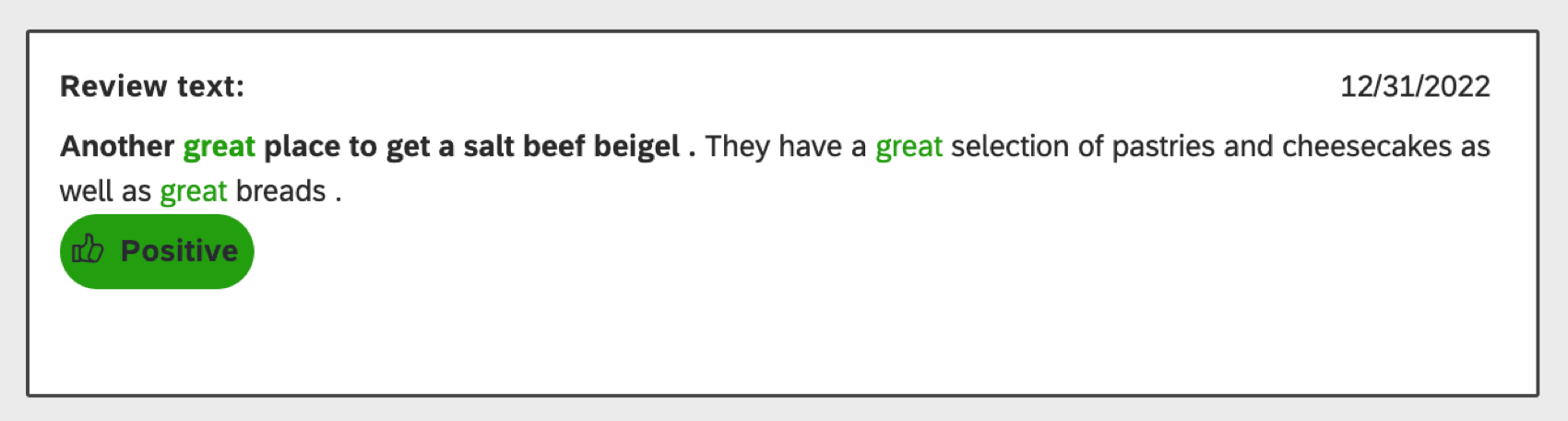
How Sentiment Is Calculated
XM Discover analyzes the sentiment of a sentence based on the tone of the words and phrases within the sentence.
- Words: Designer comes with thousands of words that are flagged as positive or negative, based on our implementation experience. You can either use default tunings or adjust them to better suit your data with tuning.
- Phrases: In addition to word-level sentiment, Designer also recognizes sentiment modifiers and exception rules that cover most well-known sentiment-changing linguistic patterns for all supported languages. You can manage default rules or create custom ones.
- Machine Learning Sentiment: Discover uses machine learning to evaluate the positivity/negativity of text and assign sentiment labels to sentences across all supported languages. If it is enabled, this kind of sentiment replaces the rules-based approaches for brands.
Sentiment Modifiers
Sentiment modifiers are additional words that affect a sentiment word’s value. They can increase, decrease, or invert the original word’s sentiment value. These words are:
- Very: Increases sentiment value by 1, towards the extreme ends of the scale.
Example: “The food was good” has a sentiment score of +2, while “The food was very good” has a sentiment score of +3. Alternatively, “The food was bad” has a sentiment score of -2 while “The food was very bad” has a sentiment score of -3.
- Slightly: Decreases sentiment value by 1, towards the middle of the scale.
Example: “The service was good” has a sentiment score of +2 while “The service was slightly good” has a sentiment score of +1. Alternatively, “The service was bad” has a sentiment score of -2 while “The service was slightly bad” has a sentiment score of -1.
- Nothing: Inverts the sentiment value.
Example: “There was nothing bad about my experience” has a sentiment score of +2 (since “bad” is normally -2), while “I have nothing good to say” has a sentiment score of -2 (since “good” is normally +2).
Sentiment Scale
When adding sentiment to widgets, you can often choose between a 3 and 5 point scale. The 3 point scale only includes negative, neutral, and positive.
>Scale Segment | >Default Range | >Default Color |
>Strongly Negative | >less than –2.5 | >Dark red |
>Negative | >between –2.5 and –1 | >Red |
>Neutral | >between –1 and 1 | >Gray |
>Positive | >between 1 and 2.5 | >Green |
>Strongly Positive | >greater than 2.5 | >Dark green |
Example Sentence Sentiment Calculations
This section provides several examples of how sentence sentiment is calculated. The overall sentence sentiment is a function of the individual sentiment scores of each sentiment word in the sentence.
Single Sentiment Word
If a sentence contains a single sentiment word, the sentence sentiment will equal the word sentiment.
Multiple Sentiment Words
If a sentence contains multiple sentiment words, then the overall sentence sentiment is calculated as follows:
- The word with the highest sentiment value in the sentence is identified. This is used as a base for the sentence sentiment.
- Then, 0.5 points are added for every additional word with the same sentiment.
- Then, 0.25 points are added for every word one level lower in sentiment.
- For each subsequent sentiment level (n-1) the system takes the points from one level above (n), divides them by 2, and then multiplies by the number of words with sentiment n-1.
Qtip: The only exception is that words with a sentiment level of 0.25 (multiple decreasing modifiers attached to the word with a +1 or –1 value) are treated the same way as sentiment levels 0.5—the net effect for the sentence sentiment will be the same for both levels as there is no meaningful difference between the two cases.
Example: In the sentence, “The room was clean, very spacious, nicely decorated, and inexpensive,” there are 4 sentiment words. These words and their scores are:
- Clean: +3
- Spacious: +3
- Nicely: +2
- Inexpensive: +1
Using the above calculation, the sentence’s sentiment is calculated as: 3 + 0.5 + 0.25 + 0.125 = 3.875.
Handling Negative Sentiment
Negative sentiment values are handled exactly the same, except that they are subtracted (because you are adding a negative number).
Using Sentiment Data
Sentiment can be used across XM Discover to build informative dashboards. Display sentiment to your stakeholders, filter data, and even build unique custom sentiment metrics to meet your organization’s needs.
When paired with text analytics, sentiment analysis can be beneficial in many ways:
- Prioritize customer feedback and handle large volumes of it consistently and accurately.
- Discover your customers’ opinion on everything from your products and services to your location, your advertisements, or even your competitors.
- Understand what customers like and dislike about you and your brand.
- Analyze customer emotional feedback from social media, your website, your call center representatives, or any other source that contains useful business information.
- Keep up with changes in public opinion toward any aspect of your business.
- Identify where you need to make product improvements, train sales or customer care representatives, or create new marketing campaigns resulting from peaks or valleys in sentiment scores.
Visualizing Sentiment
Sentiment visualization helps you get a better idea of how the sentiment scores are applied to the actual words and sentences in your project.
Sentiment visualization highlights the following information:
- Sentiment-bearing words
- Sentiment exception rules
- Sentiment modifiers
- Overall sentence sentiment
Sentiment-Bearing Words
The sentiment calculation is based on a seed list of sentiment-bearing words.
Every word can have a sentiment score from –5 (the most negative emotion) to +5 (the most positive emotion). Sentiment score ranges are grouped into five sentiment bands. Non-neutral words are colored according to sentiment bands:
- Deep red: Very negative
- Pale red: Negative
- Pale green: Positive
- Deep green: Negative
Sentiment Exception Rules
Sentiment exception rules are used to adjust sentiment calculation for idiomatic expressions where the intended sentiment behind words differs from the norm. Words that are part of an exception rule are underlined, and the central token (the word for which sentiment score is modified by the rule) is additionally italicized.
Consider the following examples:
- “The staff was too friendly.”
- “It feels like New York.”
- “The quad-pooled jacuzzi was to die for!”
These are flagged by sentiment exception rules because:
- “Friendly” is a positive word, but too much of something positive carries a negative connotation.
- “Like” as a verb is positive, but “like” as a preposition is neutral.
- “Die” is a negative word, but not when used in the phrase “to die for.”
Sentiment Modifiers
Additionally, words for which sentiment was negated (sentiment flipped to the opposite), intensified (sentiment moved further away from neutral), or weakened (sentiment moved closer to neutral without becoming neutral as a result) are double-underlined. For example, consider the following sentence:
- “There is nothing fancy about this hotel.”
The sentiment for the word “fancy” (positive by default) is changed to negative by the preceding word “nothing.”
When a sentiment-bearing word is weakened by a modifier and becomes neutralized as a result, it appears in a gray font. For example, consider the following sentence:
- “The only downside was that breakfast was not included.”
In this case the sentiment for the word “downside” (–1 by default) is changed to neutral (0) by the preceding modifier “only.”
Overall Sentence Sentiment
The overall sentiment of a sentence takes into account its sentiment-bearing words, exception rules, and sentiment modifiers. Sentence sentiment is represented by thumb icons colored according to sentiment bands. By default, this is the same color scheme as individual sentiment words.
Grouping Widget Data by Sentiment
When creating widgets in Studio, you can group data by sentiment. When you group data by sentiment, it’s important to keep in mind the following difference between sentence-level and record-level sentiment in Studio:
- While each sentence can only have 1 sentiment score, a document has as many sentiment scores as there are sentences in it.
- That means that if a document contains 1 positive and 1 negative sentence, it is considered both a positive and a negative document.
- This is why when data is grouped by sentiment, the total count is usually less than the sum of all records in each band. See the widget below.
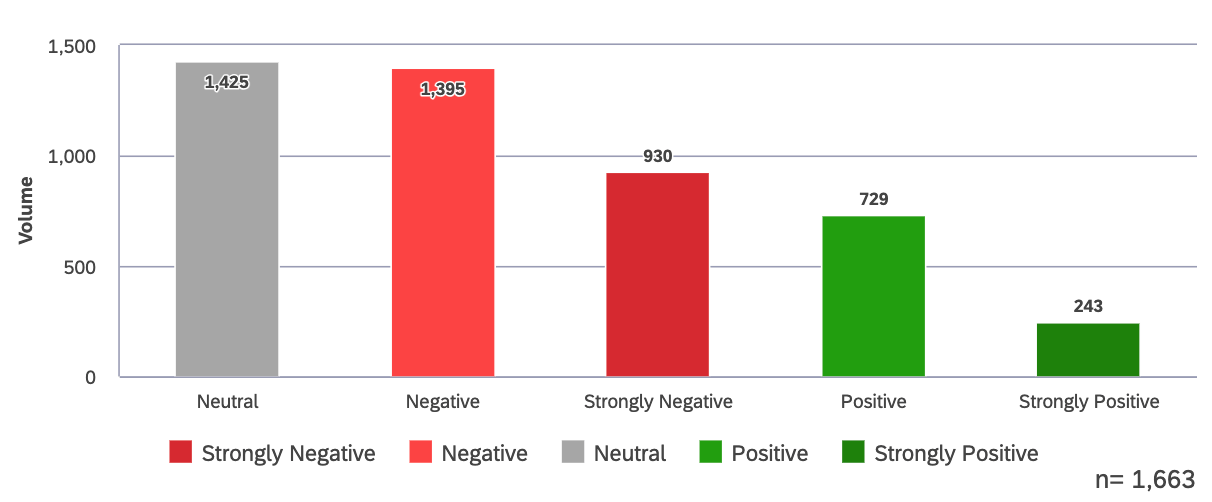
When data is grouped by sentiment, the colors in the widget will match the defaults set for the sentiment bands. Color may not match these settings if a different field is used to group data.
Machine Learning Based Sentiment
Machine learning based sentiment assigns labels ranging from “Very Negative” to “Very Positive” to sentences.
- Name: Sentiment
- System Name: XM_sentiment
- Type: Numeric
- Scale: -2 to 2
Qtip: If a sentence does not contain sentiment bearing words, it will be given the value “N/A”. The sentiment will be labeled “Neutral” when coloring by sentiment in an aggregate widget if all other sentences in the data point are also “N/A”.
- Granularity: Sentence
- Feedback Type: All
- Supported Languages: All
Rules Based Sentiment
Here’s some information on the Sentiment attribute in Designer:
- Name: CB Degree Sentiment Index
- System Name: _degreesentimentindex
- Type: Numeric
- Scale: -5 to 5
- Granularity: Sentence
- Feedback Type: All
- Supported Languages: Talk to your Discover Account Representative.