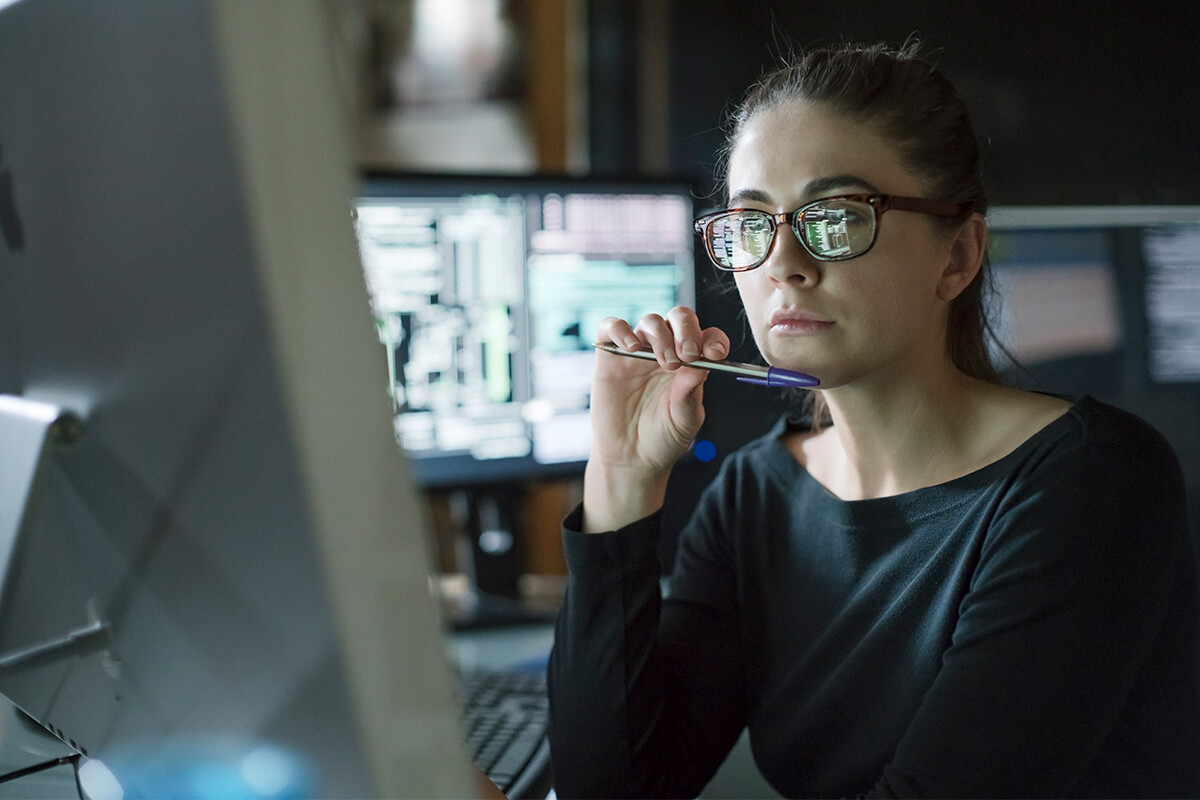
Experience Management
Synthetic responses 101 for researchers
Advances in technology have unlocked new realms of possibility for researchers to harness the power of AI like never before. One innovation that has emerged as a result is "synthetic responses”.
But what exactly are synthetic responses? How are they generated? How are traditional statistical modeling techniques different from synthetically-generated data? And what are the benefits and use cases to market researchers?
Below, we’ll unpack these concepts, highlight their differences, and explore the contexts in which they are applied.
What are synthetic responses?
Synthetic responses refer to modeled or artificially generated data that mimic the structure, characteristics, and relationships found in primary or observed data (interview, survey or operational data). These responses are modeled off of repositories of publicly available data (accessed through popular LLMs) and made even smarter and stronger by combining those sources with companies’ or brands’ proprietary intelligence (primary research data, operational data, behavioral data).
Synthetic responses are transforming data generation. By mimicking the statistical properties of data that we have, synthetic responses can empower researchers, practitioners, and businesses to derive meaningful insights while navigating challenges related to data scarcity, privacy, and ethical concerns. As methodologies and technologies for generating synthetic data continue to evolve, this innovative approach will play a pivotal role in the future of decision-making.
Rather than simply enhancing existing datasets through methods like imputation, synthetic responses generate completely new observations that statistically reflect the populations of interest. This approach allows researchers, data analysts, and machine learning practitioners to expand datasets, enhance privacy, or simulate data in scenarios where primary data is limited or difficult to obtain.
Traditional statistical techniques explained
Before delving into the differences, let’s briefly explain the traditional statistical techniques that are often employed:
- Imputation: Replacing missing values in a dataset with substituted values. Imputation can be simple (like using the mean, median, or mode) or complex (employing predictive models). The aim is to fill gaps in the data to enable robust analyses without losing valuable information.
- Extrapolation: The process of estimating unknown values by extending the trend of known data points. For example, if you have a dataset of sales figures for the past three years, you might extrapolate to predict sales for the upcoming year.
- Weighting: Adjusting the influence of certain observations in a dataset. This is often utilized in survey data analysis to ensure that the sample reflects the population accurately. In this method, a weight is assigned to each observation based on its importance or representation.
The differences between synthetic responses and traditional statistical techniques
Synthetic responses are proactive in generating completely new data points or observations about a population and/or a topic. Meanwhile, traditional techniques like imputation, extrapolation, and weighting require existing data to manipulate or estimate values.
The appeal of synthetic responses is to generate data around a topic that the inquirer doesn’t have insight into today, and secondarily to expand or build upon existing insights or datasets, honoring the population distributions and known details in the primary or observed data. Conversely, traditional techniques are typically aimed at making adjustments or estimations to maximize the utility of incomplete or unrepresentative datasets.
Synthetic responses are particularly useful in scenarios with sensitive data (e.g., health records, personal information) where privacy concerns necessitate non-identifiable data. Traditional methods, such as imputation and extrapolation, focus primarily on data integrity when dealing with missing values or making forecasts.
Traditional techniques can sometimes lead to biased results if not carefully applied; for instance, naïve imputation methods can distort existing distributions. Synthetic responses, when properly generated, can reduce bias by incorporating diverse patterns from the primary or observed data without directly copying from it.
The choice between synthetic responses and traditional techniques is highly context-dependent. If you're looking to test an idea, explore a new market or are dealing with privacy-sensitive information, synthetic responses may be ideal. In contrast, if your primary concern is managing missing data or matching population distributions within your existing dataset, traditional techniques would be more appropriate.
Benefits of synthetic responses in market research
Generating synthetic data is also often faster and more cost-effective than collecting real-world data, allowing organizations to make decisions with confidence and accelerate their time-to-market. Robust traditional market research studies can take upwards of 8-10 weeks before insights are shared; synthetic data reduces this to hours.
According to our 2025 market research trends report, the top 5 advantages of using synthetic responses are:
Companies go to great lengths to protect intellectual property, but when testing new products, services, or features, it can be challenging to get the impactful data you need without taking on substantial risk. Synthetic data can bridge information gaps by acting as substitutes for real data. This prevents sensitive information like personal details and intellectual property from being revealed and does so in a way that is quicker and cost-effective.
Lastly, synthetic responses give researchers a way to reach more diverse populations
by simulating a wide range of demographic profiles. These might be underrepresented populations in our traditional or human-based data collection or audiences that are traditionally harder to reach.
Use cases and applications for synthetic responses
Global market researchers are using synthetic data in a number of ways. The top 2 use cases researchers are leveraging synthetic responses for include user experience and early stage innovation (think idea generation, idea screening, early stage concept testing, concept optimization, and feature prioritization). Researchers frequently need to understand how changes in product attributes, pricing, or marketing strategies might affect consumer behavior; synthetic responses can test the impact of these factors, model outcomes and reduce risk before launching.
Other common use cases researchers consider synthetic a good fit for include brand research (brand health tracking, brand perception, positioning), pricing research, foundational research (attitude and usage, segmentations, market landscape), go-to-market research (package testing, naming testing, claims/message testing) and product satisfaction post-launch. To break into new markets and audiences, researchers can create synthetic datasets that reflect various market segments based on demographic, psychographic, and behavioral characteristics. These communities of simulated audiences then allow researchers to analyze preferences and needs of this new demographic based on related data from existing market segments. This proactive approach enables tailored marketing strategies that are more likely to resonate with specific consumer groups.
Beyond these common use cases, there are a wide range of applications across various fields, including:
Pre-testing survey design: Building effective surveys requires understanding the types of questions that resonate with respondents and yield valuable data.
Synthetic data can be generated to simulate responses to proposed survey questions. By analyzing these simulated responses, researchers can identify potential biases, misunderstandings, or areas for improvement in survey design. This iterative process can enhance the overall efficacy of surveys and the quality of insights they provide.
Mitigating bias in data collection: Traditional methods of data collection can introduce biases that misrepresent consumer behavior, such as those stemming from non-response or sample selection bias. By using synthetic data that reflects the overall population more accurately, researchers can reduce biases inherent in their existing datasets. For example, if certain demographics are underrepresented in a survey about product usage, synthetic data can be generated to reflect a more balanced view. This correction helps ensure that analytical insights are robust, leading to more accurate market assessments and strategy development.
Protecting sensitive information: Market researchers often collect (or expose) sensitive information, which raises ethical and privacy concerns.
Utilizing synthetic data can help protect individual consumer identities while still providing viable datasets for analysis. For instance, in studies involving health-related products or personal financial data, using synthetic responses allows researchers to analyze trends and preferences without compromising privacy. Companies can present findings and trends based on synthetic data while ensuring that no real individual’s data is disclosed.
Things to consider as adoption of synthetic responses rises:
- Quality Assurance: It's essential to ensure that synthetic data accurately reflects the properties of the real data. Poorly generated synthetic data can lead to misleading conclusions.
- Overfitting: When using synthetic data for training machine learning models, there is a risk of models overfitting to the synthetic patterns instead of generalizing to real-world scenarios.
- Ethical Considerations: Care must be taken to ensure that synthetic data does not inadvertently reinforce biases present in the original dataset or risk / violate trust with the consumer populations that the data is modeled after.
Qualtrics’ approach to synthetic data
Synthetic responses represent an exciting frontier in data analysis, enabling researchers and practitioners to broaden their datasets while addressing some of the limitations associated with traditional statistical methods. By understanding the differences and applications of synthetic responses as compared to imputation, extrapolation, and weighting, you can make informed decisions about the best strategies for your data analysis needs. As technology continues to evolve, the integration of synthetic responses into analytical pipelines could redefine how we approach and engage with data across various domains.
Qualtrics Edge helps companies make the right call when it matters most through insights subscriptions and bespoke engagements. We empower clients by arming them with a powerful set of AI-powered solutions that provide instant insights.
By providing always-on intelligence to power breakthrough decisions and uncover overnight opportunities Qualtrics Edge is transforming the way research is done with offerings like connected intelligence, simulated audiences and market activity predictions. We exist to connect the dots between what consumers think and how they act, so we can predict what they might do next. Our industry-leading research expertise, run on a world-class experience platform, enhanced with unique data, leads to faster, richer and more reliable outcomes.
Take your research to the next level with XM for Strategy & Research