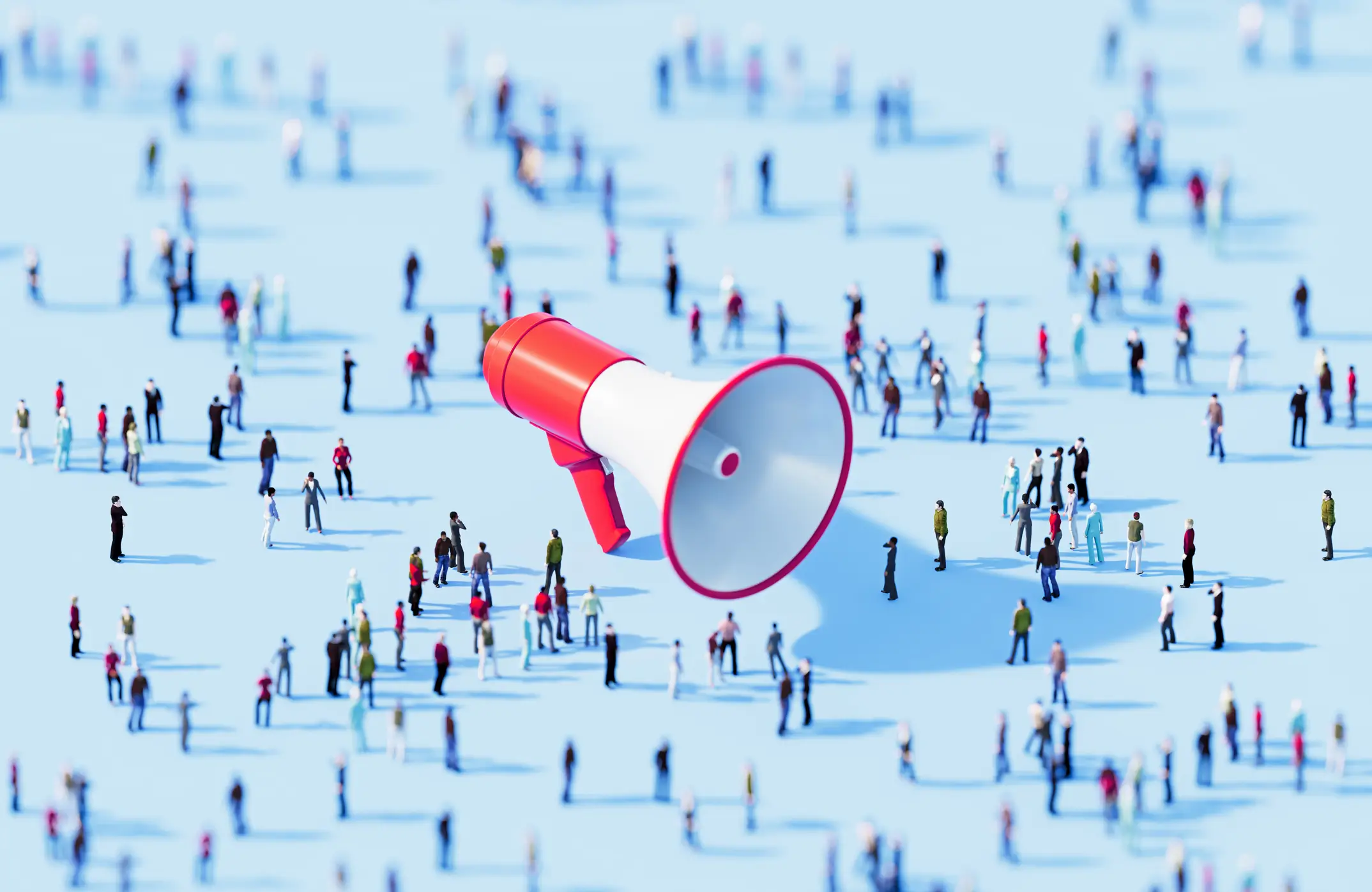
Brand Experience
How to build AI brand tracking strategies
Brand health tracking has evolved from a periodic checkup to a mission-critical intelligence system for forward-thinking companies. By monitoring brand awareness, sentiment, and loyalty, organizations gain the insights needed to make strategic decisions that drive growth and consumer connection.
Let's explore how modern brand health tracking, especially when enhanced with AI, can transform your marketing strategy.
Key takeaways
- Brand health tracking provides essential data for monitoring performance, understanding consumer perception, and enabling data-driven marketing decisions.
- Key metrics including Net Promoter Score (NPS), brand awareness, and brand sentiment create a comprehensive picture of overall brand health and market position.
- AI has revolutionized brand health tracking by delivering real-time insights and predictive analytics, allowing brands to pivot strategies before problems arise.
A quick recap of brand health tracking
Brand tracking measures how your brand performs in consumers' minds and hearts over time. This continuous assessment gives you visibility into how people perceive your brand, how effectively your marketing resonates, and the tangible return on investment your brand-building efforts generate.
What you measure, how customers engage with your brand, and shifts in brand sentiment all influence the insights you'll gain. The right frequency for your brand tracking depends on your specific goals, resources, and market dynamics – there's no one-size-fits-all approach to tracking your brand.
Free eBook: The ultimate guide to building a world-class brand tracker
The role of AI in brand health tracking
Artificial intelligence has fundamentally transformed how brands monitor and interpret health metrics. Traditional methods often struggled with the scale and complexity of modern data streams, but AI-powered tools now provide real-time optimization capabilities and predictive insights that were previously impossible.
Revolutionizing data analysis and pattern detection
AI-driven brand health monitoring leverages machine learning algorithms to analyze vast amounts of qualitative and quantitative data simultaneously, detecting patterns and anomalies that human analysts might miss. These sophisticated systems connect previously disparate data points to reveal hidden correlations between brand metrics and business outcomes, enabling marketers to make strategic adjustments with unprecedented speed and precision.
Enhancing consumer understanding through NLP
Natural Language Processing (NLP) algorithms efficiently analyze thousands of open-ended survey responses, uncovering nuanced consumer perceptions that would take human analysts weeks to identify. This capability transforms qualitative data into quantifiable insights without losing the richness of customer voice, creating a more accurate picture of brand positioning and sentiment.
Driving hyper-personalization at scale
AI enables hyper-personalization by tailoring messages to individual consumers based on browsing history, purchase behavior, and engagement patterns. Predictive models identify which personalization approaches are most likely to resonate with specific audience segments, dramatically improving conversion rates and strengthening brand relationships across digital touchpoints.
Creating seamless information flow across organizations
Integration of AI tools with existing marketing systems creates a seamless flow of information across the organization. When brand health data connects with customer experience platforms, marketing automation systems, and sales intelligence tools, the entire company can align around consumer insights. This unified approach ensures that brand tracking isn't just a measurement exercise but a strategic driver of business decisions.
Providing competitive intelligence in real-time
AI-driven competitive analysis provides sophisticated understanding of market positioning and share of voice. By continuously monitoring competitors' messaging, sentiment, and customer reactions in real time, brands can identify opportunities and threats before they become obvious to the rest of the market. Machine learning models can even predict competitors' likely next moves based on historical patterns and current market signals.
Balancing AI insights with human expertise
The most effective brand health strategies balance AI-generated insights with human expertise. While algorithms excel at pattern recognition and data processing, experienced marketers provide critical context, creativity, and strategic direction that machines cannot replicate. Leading organizations pair AI brand research systems with cross-functional teams that can translate technical insights into meaningful brand initiatives.
As AI technology continues to advance, its role in brand health tracking will expand further, offering even more sophisticated tools for anticipating consumer needs and market shifts. Organizations that embrace these technologies now will develop the institutional knowledge and data infrastructure needed to maintain competitive advantage in an increasingly dynamic marketplace.
Key metrics for measuring brand health with AI
Effective AI-driven brand health measurement leverages predictive analytics to monitor key metrics that reveal both immediate customer perceptions and forecast future market performance. These intelligent indicators serve as your brand's early warning system, using pattern recognition to detect potential issues before they materialize. Three essential metrics form the foundation of any AI-powered brand health tracking strategy:
Net Promoter Score (NPS)
AI has transformed NPS from a simple survey metric into a predictive powerhouse for measuring customer loyalty and satisfaction. Machine learning algorithms now analyze NPS responses alongside behavioral data to identify which detractors are at risk of defection and which promoters might become brand advocates. The calculation remains straightforward—subtract detractors (0-6) from promoters (9-10)—but AI enriches this data with contextual understanding.
Sentiment analysis algorithms can now detect nuanced emotional signals in NPS verbatim comments, allowing brands to understand not just the score but the complex feelings driving customer responses. AI-driven benchmarking also provides more sophisticated competitive context, showing how your NPS performance compares against industry peers in real time rather than through periodic reports.
Brand awareness
AI-enhanced brand awareness measurement combines traditional survey methods with digital footprint analysis to create a comprehensive view of recognition across channels. Advanced NLP monitors social media conversations, search behavior, and online forums to gauge unprompted brand mentions, complementing explicit measurement through aided and unaided recall surveys.
Machine learning algorithms now detect subtle patterns in awareness data, identifying which customer segments show early recognition growth and which might be at risk of decline. Computer vision technology can even assess brand recognition in visual contexts, measuring how consumers respond to logos, colors, and brand imagery across digital touchpoints.
Predictive modeling using multiple AI engines provides more reliable awareness forecasting, helping brands understand not just current recognition levels but future trajectories based on marketing activities and competitive movements.
Brand sentiment
AI has completely transformed brand sentiment analysis through sophisticated emotion detection algorithms that identify complex feeling states beyond simple positive/negative classifications. These systems analyze linguistic patterns, emoji usage, image content, and even audio characteristics to create multi-dimensional sentiment profiles.
Real-time AI brand monitoring tools continuously scan social media platforms, review sites, customer service interactions, and other digital touchpoints, alerting brands to emerging sentiment shifts before they become widespread. Machine learning models identify sentiment patterns specific to your industry and brand, recognizing specialized terminology and context that generic analysis might miss.
A leading electronics manufacturer leveraged AI-powered sentiment analysis to detect subtle dissatisfaction with a new product feature within days of launch. The system identified specific language patterns indicating confusion rather than outright dissatisfaction, allowing the company to deploy targeted educational content rather than a costly product modification.
Benefits of continuous brand health monitoring
AI-powered continuous brand health monitoring serves as a predictive intelligence system, using machine learning algorithms to identify potential issues before traditional metrics would detect them. This proactive approach prevents market share erosion by forecasting declines in brand awareness or sentiment with remarkable accuracy, often 3-6 months earlier than conventional methods.
Automated real-time monitoring enables instantaneous response to sentiment shifts, with AI systems not only detecting changes but recommending specific interventions based on historical success patterns. Some advanced platforms can even automate responses to common sentiment triggers, adjusting digital advertising messaging or social media content without human intervention when certain thresholds are crossed.
Predictive analytics within AI brand monitoring systems continuously analyze emerging customer needs by identifying nascent conversation patterns across digital channels. These systems can detect subtle linguistic shifts that indicate evolving preferences, giving brands crucial intelligence for product development and positioning. By leveraging natural language processing to analyze thousands of conversations simultaneously, AI tools provide competitive intelligence at a scale and speed impossible with human analysis alone.
Building an effective, AI-powered brand health tracking strategy
Creating a powerful AI-driven brand health tracking strategy begins with defining clear objectives that machine learning systems can optimize toward. Whether you aim to increase brand awareness, improve sentiment, or drive purchase intent, modern AI platforms can automatically adjust measurement parameters to focus on the most predictive indicators for your specific goals.
Several factors influence the optimal configuration of AI brand tracking systems, including market volatility, competitive activity, and campaign schedules. While traditional brand trackers operated on fixed schedules, AI-powered systems can dynamically adjust measurement frequency based on detected anomalies or opportunities, intensifying data collection during critical periods.
Setting objectives and KPIs
AI transforms objective-setting by identifying which brand metrics most strongly correlate with business outcomes in your specific industry. These intelligent systems analyze historical data to determine which KPIs serve as leading indicators for sales, customer retention, and market share growth.
Machine learning algorithms can develop comprehensive measurement frameworks that account for multiple dimensions of brand health simultaneously. Rather than focusing on isolated metrics, these systems create interconnected models that reflect the complex relationship between awareness, consideration, preference, and advocacy.
Selecting the right tools
The AI brand tracking landscape includes sophisticated options ranging from predictive analytics platforms to real-time sentiment monitoring systems and automated competitive intelligence tools. When evaluating these technologies, consider their machine learning capabilities, data integration features, and ability to deliver actionable insights rather than just raw data.
Leading AI platforms now offer pre-built industry models trained on relevant category data, dramatically reducing implementation time while improving accuracy. Look for systems that combine multiple AI technologies—including natural language processing, computer vision, and predictive analytics—to create a comprehensive brand intelligence ecosystem.
Data collection and analysis
AI has revolutionized data collection through automated multi-channel listening posts that continuously gather brand mentions across digital touchpoints. These systems use sophisticated entity recognition to identify relevant conversations even when brand names aren't explicitly mentioned, capturing indirect references that traditional monitoring would miss.
Machine learning algorithms automatically validate and clean incoming data, identifying anomalies that might indicate quality issues without human intervention. Advanced AI systems can even synthesize insights across multiple data sources, creating unified brand health assessments that combine survey data, social listening, sales information, and competitive intelligence into coherent strategic recommendations.
Frequently asked questions about brand health tracking
What factors influence the frequency of running a brand tracker?
The optimal frequency depends on market volatility, competitive landscape changes, advertising campaign schedules, and unexpected events that might impact consumer perception. Most companies conduct brand tracking annually or semi-annually, with additional pulse checks during significant market developments.
What is the purpose of brand tracking?
Brand tracking collects and analyzes consumer feedback to understand preferences, perceptions, and behaviors related to your brand. This information enables companies to enhance products or services, adjust marketing strategies, and maintain competitive advantage.
What should an effective brand tracker accomplish?
An effective brand tracker should monitor performance metrics over time, adapt to changing market conditions, provide clear actionable insights, and deliver regular reporting that informs strategic decision-making across the organization.
What is the significance of consumer perceptions of a brand?
Consumer perceptions directly influence trust, pricing power, employee attraction, and ultimately business performance. Understanding these perceptions helps companies tailor strategies to strengthen positive associations and address negative ones before they impact the bottom line.
How is AI transforming brand health tracking?
AI transforms brand health tracking through real-time monitoring, predictive analytics, automated sentiment analysis, and the ability to process vast amounts of unstructured data. These capabilities enable more timely insights, deeper understanding of consumer emotions, and more accurate forecasting of brand performance.
Common challenges and solutions in brand health tracking
Despite its transformative potential, AI-powered brand health tracking presents several common implementation challenges. Data quality concerns remain paramount, with machine learning systems requiring clean, consistent inputs to generate reliable insights. A global telecommunications provider addressed this by implementing AI-driven data validation that automatically flags statistically anomalous responses and recommends appropriate corrective actions.
Free eBook: The ultimate guide to building a world-class brand tracker
Ensuring data quality and consistency
Data quality concerns remain paramount, with machine learning systems requiring clean, consistent inputs to generate reliable insights. A global telecommunications provider addressed this by implementing AI-driven data validation that automatically flags statistically anomalous responses and recommends appropriate corrective actions.
Overcoming integration challenges
Integration difficulties between legacy measurement systems and new AI platforms often create technical bottlenecks. One solution involves implementing API-based middleware that translates data between systems, allowing organizations to preserve historical tracking data while adding AI capabilities. A major retailer successfully adopted this approach, maintaining decade-long trend data while enhancing analysis with predictive algorithms.
Addressing algorithm transparency
Algorithm transparency represents another significant challenge, as "black box" AI models may generate insights without clear explanations of their reasoning. Leading brand tracking platforms now incorporate explainable AI technologies that provide natural language summaries of analytical pathways, helping marketers understand not just what is happening with their brand but why the AI system reached specific conclusions.
Managing data privacy concerns
Data privacy concerns have intensified as AI systems collect increasingly granular information about consumer behavior and preferences. Successful implementations address these concerns through privacy-preserving analytics techniques like differential privacy and federated learning, which extract insights without exposing individual-level data. These approaches satisfy regulatory requirements while still delivering the predictive power of AI-enhanced brand tracking.
Navigating organizational resistance
Resistance to automation often emerges from marketing teams accustomed to traditional brand tracking methodologies. Organizations can overcome this challenge through phased implementation approaches that gradually introduce AI capabilities alongside familiar metrics and reporting formats. This creates confidence in the new systems while demonstrating concrete value through superior predictive accuracy and faster insights generation.
Future trends in AI-powered brand health tracking
The future of brand health tracking looks increasingly intelligent and integrated. Emerging technologies like predictive analytics will enable brands to forecast potential issues and opportunities before they materialize. These capabilities will transform brand tracking from a monitoring tool to a strategic planning asset.
Multi-modal analysis that combines text, visual, audio, and behavioral data will provide unprecedented insights into brand perception across different contexts and touchpoints. This holistic view will help brands understand the complete consumer experience rather than fragmented interactions.
Real-time decision support systems will connect brand health data directly to marketing activation platforms, enabling immediate optimization of campaigns based on consumer response. This closed-loop approach will dramatically improve marketing efficiency and effectiveness.
How Qualtrics AI can help track your brand’s health
Mastering brand health tracking represents a significant competitive advantage in today's dynamic marketplace. By understanding key metrics, leveraging AI tools, and implementing continuous monitoring, organizations can make informed decisions that strengthen brand equity and drive business growth.
Building effective AI brand tracking strategies requires clear objectives, appropriate tools, and comprehensive data collection methods. With these elements in place, companies can gain actionable insights that inform everything from product development to marketing communications.
Advanced solutions like Qualtrics® Brand & Communication provide sophisticated analytics and real-time insights that transform brand tracking from a retrospective assessment into a forward-looking strategic advantage. As AI capabilities continue to evolve, brand health tracking will become even more powerful, helping organizations build stronger connections with consumers and achieve sustainable growth.
Free eBook: The ultimate guide to building a world-class brand tracker